



Date:20/12/17
Microscopes enhanced with artificial intelligence (AI) could help clinical microbiologists diagnose potentially deadly blood infections and improve patients’ odds of survival, according to microbiologists at Beth Israel Deaconess Medical Center (BIDMC). In a paper published in the Journal of Clinical Microbiology, the scientists demonstrated that an automated AI-enhanced microscope system is “highly adept” at identifying images of bacteria quickly and accurately. The automated system could help alleviate the current lack of highly trained microbiologists, expected to worsen as 20 percent of technologists reach retirement age in the next five years.
“This marks the first demonstration of machine learning in the diagnostic area,” said senior author James Kirby, MD, Director of the Clinical Microbiology Laboratory at BIDMC and Associate Professor of Pathology at Harvard Medical School. “With further development, we believe this technology could form the basis of a future diagnostic platform that augments the capabilities of clinical laboratories, ultimately speeding the delivery of patient care.”
Kirby’s team used an automated microscope designed to collect high-resolution image data from microscopic slides. In this case, blood samples taken from patients with suspected bloodstream infections were incubated to increase bacterial numbers. Then, slides were prepared by placing a drop of blood on a glass slide and stained with dye to make the bacterial cell structures more visible.
Next, they trained a convolutional neural network (CNN) – a class of artificial intelligence modeled on the mammalian visual cortex and used to analyze visual data – to categorize bacteria based on their shape and distribution. These characteristics were selected to represent bacteria that most often cause bloodstream infections; the rod-shaped bacteria including E. coli; the round clusters of Staphylococcus species; and the pairs or chains of Streptococcus species.
“Like a child, the system needed training,” said Kirby. “Learning to recognize bacteria required a lot of practice, making mistakes and learning from those errors.”
To train it, the scientists fed their unschooled neural network more than 25,000 images from blood samples prepared during routine clinical workups. By cropping these images – in which the bacteria had already been identified by human clinical microbiologists – the researchers generated more than 100,000 training images. The machine intelligence learned how to sort the images into the three categories of bacteria (rod-shaped, round clusters, and round chains or pairs), ultimately achieving nearly 95 percent accuracy.
Next, the team challenged the algorithm to sort new images from 189 slides without human intervention. Overall, the algorithm achieved more than 93 percent accuracy in all three categories. With further development and training, Kirby and colleagues suggest the AI-enhanced platform could be used as fully automated classification system in the future.
In the meantime, Kirby suggests automated classification can ameliorate the shortage of human technologists by helping them work more efficiently, “conceivably reducing technologist read time from minutes to seconds,” he said.
While human technologists routinely provide highly accurate diagnoses, demand for these highly skilled workers exceeds supply in the United States. Nine percent of lab technologists remain unfilled, and that number is expected to dramatically increase as technologists of the Baby Boomer generation begin to retire in droves, according to a 2014 survey from the American Society for Clinical Pathology.
What’s more, these images can be sent remotely, bringing the highest level expertise anywhere the internet reaches. That’s critical, as rapid identification and delivery of antibiotic medications is the key to treating bloodstream infections, which can kill up to 40 percent of patients who develop them. Each day a patient goes untreated is linked with an increased risk of mortality.
In addition to its clinical uses, the new tool could also have applications in microbiology training and research, Kirby noted.
“The tool becomes a living data repository as we use it,” he said. “And could be used to train new staff and ensure competency. It can provide unprecedented level of detail as a research tool.”
In addition to Kirby, study authors include co-lead authors, postdoctoral fellow, Kenneth P. Smith, Ph.D., and, medical microbiology fellow, Anthony D. Kang, Ph.D., both members of the Department of Pathology at BIDMC.
This work was supported by the Harvard Clinical and Translational Science Center (National Center for Research Recourses and the National Center for Advancing Translational Science, National Institutes of Health Award UL1 TR001102) and by financial contributions from Harvard University and its affiliated academic healthcare centers. Anthony Kang was supported by the United States Army’s Long Term Health Education and Training program as an American Society for Microbiology Committee on Postgraduate Educational Programs Fellow at BIDMC. Kenneth Smith was supported by the National Institute of Allergy and Infectious Diseases of the National Institutes of Health (F32 AI124590).
BIDMC Researchers Use Artificial Intelligence to Identify Bacteria Quickly and Accurately
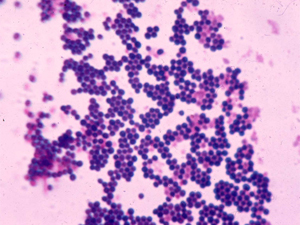
“This marks the first demonstration of machine learning in the diagnostic area,” said senior author James Kirby, MD, Director of the Clinical Microbiology Laboratory at BIDMC and Associate Professor of Pathology at Harvard Medical School. “With further development, we believe this technology could form the basis of a future diagnostic platform that augments the capabilities of clinical laboratories, ultimately speeding the delivery of patient care.”
Kirby’s team used an automated microscope designed to collect high-resolution image data from microscopic slides. In this case, blood samples taken from patients with suspected bloodstream infections were incubated to increase bacterial numbers. Then, slides were prepared by placing a drop of blood on a glass slide and stained with dye to make the bacterial cell structures more visible.
Next, they trained a convolutional neural network (CNN) – a class of artificial intelligence modeled on the mammalian visual cortex and used to analyze visual data – to categorize bacteria based on their shape and distribution. These characteristics were selected to represent bacteria that most often cause bloodstream infections; the rod-shaped bacteria including E. coli; the round clusters of Staphylococcus species; and the pairs or chains of Streptococcus species.
“Like a child, the system needed training,” said Kirby. “Learning to recognize bacteria required a lot of practice, making mistakes and learning from those errors.”
To train it, the scientists fed their unschooled neural network more than 25,000 images from blood samples prepared during routine clinical workups. By cropping these images – in which the bacteria had already been identified by human clinical microbiologists – the researchers generated more than 100,000 training images. The machine intelligence learned how to sort the images into the three categories of bacteria (rod-shaped, round clusters, and round chains or pairs), ultimately achieving nearly 95 percent accuracy.
Next, the team challenged the algorithm to sort new images from 189 slides without human intervention. Overall, the algorithm achieved more than 93 percent accuracy in all three categories. With further development and training, Kirby and colleagues suggest the AI-enhanced platform could be used as fully automated classification system in the future.
In the meantime, Kirby suggests automated classification can ameliorate the shortage of human technologists by helping them work more efficiently, “conceivably reducing technologist read time from minutes to seconds,” he said.
While human technologists routinely provide highly accurate diagnoses, demand for these highly skilled workers exceeds supply in the United States. Nine percent of lab technologists remain unfilled, and that number is expected to dramatically increase as technologists of the Baby Boomer generation begin to retire in droves, according to a 2014 survey from the American Society for Clinical Pathology.
What’s more, these images can be sent remotely, bringing the highest level expertise anywhere the internet reaches. That’s critical, as rapid identification and delivery of antibiotic medications is the key to treating bloodstream infections, which can kill up to 40 percent of patients who develop them. Each day a patient goes untreated is linked with an increased risk of mortality.
In addition to its clinical uses, the new tool could also have applications in microbiology training and research, Kirby noted.
“The tool becomes a living data repository as we use it,” he said. “And could be used to train new staff and ensure competency. It can provide unprecedented level of detail as a research tool.”
In addition to Kirby, study authors include co-lead authors, postdoctoral fellow, Kenneth P. Smith, Ph.D., and, medical microbiology fellow, Anthony D. Kang, Ph.D., both members of the Department of Pathology at BIDMC.
This work was supported by the Harvard Clinical and Translational Science Center (National Center for Research Recourses and the National Center for Advancing Translational Science, National Institutes of Health Award UL1 TR001102) and by financial contributions from Harvard University and its affiliated academic healthcare centers. Anthony Kang was supported by the United States Army’s Long Term Health Education and Training program as an American Society for Microbiology Committee on Postgraduate Educational Programs Fellow at BIDMC. Kenneth Smith was supported by the National Institute of Allergy and Infectious Diseases of the National Institutes of Health (F32 AI124590).
Views: 509
©ictnews.az. All rights reserved.Similar news
- Cellphone Use May Raise Cancer Risk
- Australian police pushes cyber safety education
- Vietnam aims to lead in e-government
- Senate Website Gets Hacked
- US builds net for cyber war games
- Japan enacts anti-computer virus law
- India passes law vs e-waste
- Anonymous Declares War On The City Of Orlando
- Microsoft highlights evolving dangers as online identity data proliferates
- Consumers want internet security to be provided by banks
- Government facilities targets of cyber attack
- South Korean web attacks might been war drill
- Sri Lanka to Establish National Passport Database to Increase Border Security
- Hi-tech crime agencies set to employ information security professionals
- Phone hacking and online campaign bring down the News of the World