



Date:07/10/16
Crop diseases, which threaten the world’s food security, can be fought with the help of artificial intelligence systems. Scientists from EPFL and Penn State University have trained a deep-learning neural network that can accurately diagnose crop diseases by “seeing” and analyzing normal photographs of individual plants. The algorithm, which is part of the “PlantVillage” project, represents the first successful proof of concept for disease diagnosis through smartphone photos, and will be used to build an app for farmers. The work has been published in Frontiers in Plant Science.
The unprecedented growth of the world’s population means food shortage and ecosystem pressure will become global problems in the coming decades. PlantVillage, a project that employs algorithms to train computers to diagnose crop disease, is the brainchild of Marcel Salathé at EPFL and David Hughes at Penn State. The algorithm development itself is led by computer scientist Sharada P. Mohanty, a PhD student in Salathé’s Laboratory of Digital Epidemiology.
The project benefits from the progress that has been made in the field of “deep learning” in recent years. Deep learning is a type of machine learning that uses algorithms to find patterns in big sets of data – in this case, over 50,000 digital photographs of diseased plants, made openly available by PlantVillage. Through a computational neural network, the system processes the photographs through multiple layers of artificial neurons, and so gradually “learns” to identify different diseases with high degrees of certainty.
The goal is to put the tool in the hands of farmers, agriculturists, and everyday gardeners in the form of a smartphone app. “People will be able to snap a photograph of their sick plant with the app and get a diagnosis within seconds,” says Salathé.
To enable anyone in the world to develop such algorithms, the scientists made their database of over 50,000 photographs openly available in 2015. The current paper demonstrates their deep-learning algorithm at work: The researchers assigned every one of 54,306 photographs of diseased and healthy plant leaves to one of 38 classes of crop-disease pairs (e.g. Tomato plant-Tomato Early Blight, Apple tree – Apple scab etc).
They then trained their “deep convolutional neural network” to identify plants and diseases (or lack thereof for healthy plants), and they measured how accurately it could assign each image to the correct class. In total, working with 14 crop species and 26 different plant diseases, the system could identify diseases on images it had never seen before with an accuracy of 99.35%.
Building the algorithm and training the model require significant computing power and time, but once trained, the classification task itself is very fast, and the resulting code is small enough to be easily installed on a smartphone. "This presents a clear path towards smartphone-assisted crop-disease diagnosis on a massive global scale,” says lead author Sharada Mohanty.
However, these photographs were taken under controlled conditions of lighting, color etc, which don’t always correspond to a snapshot taken in a field. To address this, the team is now expanding their database of images to about 150,000 in order to improve the system’s ability to identify diseases. In addition, they are planning to also expand the amount of data that the network will use to make accurate diagnoses.
“At this point, we’re relying on a photograph taken by a user in a field under natural conditions,” explains Salathé. “But in the future, we would like to also bring in time, location, epidemiological trends, weather conditions and other signals to bear upon the network, which would vastly improve its abilities.” Although this system aims to supplement rather than replace existing diagnostic methods, the fact that there will be over 5 billion smartphones around the world by 2020 will be a tremendous advantage.
“We do believe that the approach represents a viable additional method to help prevent yield loss," says David Hughes. "With the ever-improving number and quality of sensors on mobile devices, we consider it likely that highly accurate diagnoses via the smartphone are only a question of time."
Artificial intelligence could help farmers diagnose crop diseases
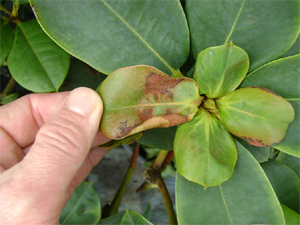
The unprecedented growth of the world’s population means food shortage and ecosystem pressure will become global problems in the coming decades. PlantVillage, a project that employs algorithms to train computers to diagnose crop disease, is the brainchild of Marcel Salathé at EPFL and David Hughes at Penn State. The algorithm development itself is led by computer scientist Sharada P. Mohanty, a PhD student in Salathé’s Laboratory of Digital Epidemiology.
The project benefits from the progress that has been made in the field of “deep learning” in recent years. Deep learning is a type of machine learning that uses algorithms to find patterns in big sets of data – in this case, over 50,000 digital photographs of diseased plants, made openly available by PlantVillage. Through a computational neural network, the system processes the photographs through multiple layers of artificial neurons, and so gradually “learns” to identify different diseases with high degrees of certainty.
The goal is to put the tool in the hands of farmers, agriculturists, and everyday gardeners in the form of a smartphone app. “People will be able to snap a photograph of their sick plant with the app and get a diagnosis within seconds,” says Salathé.
To enable anyone in the world to develop such algorithms, the scientists made their database of over 50,000 photographs openly available in 2015. The current paper demonstrates their deep-learning algorithm at work: The researchers assigned every one of 54,306 photographs of diseased and healthy plant leaves to one of 38 classes of crop-disease pairs (e.g. Tomato plant-Tomato Early Blight, Apple tree – Apple scab etc).
They then trained their “deep convolutional neural network” to identify plants and diseases (or lack thereof for healthy plants), and they measured how accurately it could assign each image to the correct class. In total, working with 14 crop species and 26 different plant diseases, the system could identify diseases on images it had never seen before with an accuracy of 99.35%.
Building the algorithm and training the model require significant computing power and time, but once trained, the classification task itself is very fast, and the resulting code is small enough to be easily installed on a smartphone. "This presents a clear path towards smartphone-assisted crop-disease diagnosis on a massive global scale,” says lead author Sharada Mohanty.
However, these photographs were taken under controlled conditions of lighting, color etc, which don’t always correspond to a snapshot taken in a field. To address this, the team is now expanding their database of images to about 150,000 in order to improve the system’s ability to identify diseases. In addition, they are planning to also expand the amount of data that the network will use to make accurate diagnoses.
“At this point, we’re relying on a photograph taken by a user in a field under natural conditions,” explains Salathé. “But in the future, we would like to also bring in time, location, epidemiological trends, weather conditions and other signals to bear upon the network, which would vastly improve its abilities.” Although this system aims to supplement rather than replace existing diagnostic methods, the fact that there will be over 5 billion smartphones around the world by 2020 will be a tremendous advantage.
“We do believe that the approach represents a viable additional method to help prevent yield loss," says David Hughes. "With the ever-improving number and quality of sensors on mobile devices, we consider it likely that highly accurate diagnoses via the smartphone are only a question of time."
Views: 361
©ictnews.az. All rights reserved.Similar news
- Azerbaijani project to monitor disease via mobile phones
- Innovative educational system to be improved under presidential decree
- NTRC prolongs license of two TV and radio organizations for 6 years
- Azerbaijan establishes e-registry for medicines
- Azerbaijani museum introduces e-guide
- Nar Mobile opens “Nar Dunyasi” sales and service center in Siyazan city
- International conference on custom electronic services held in Baku
- OIC secretary general to attend COMSTECH meeting in Baku
- Azerbaijan develops earthquake warning system
- New law to regulate transition to digital broadcasting in Azerbaijan
- Azerbaijani State Social Protection Fund introduces electronic digital signature
- Intellectual traffic management system in Baku to be commissioned in December
- Tax Ministry of Azerbaijan started receiving video-addresses
- World Bank recommends Azerbaijan to speed up e-service introduction in real estate
- Azerbaijan to shift to electronic registration of real estate