



Date:11/10/16
New research, led by the University of Southampton, has demonstrated that a nanoscale device, called a memristor, could be used to power artificial systems that can mimic the human brain.
Artificial neural networks (ANNs) exhibit learning abilities and can perform tasks which are difficult for conventional computing systems, such as pattern recognition, on-line learning and classification. Practical ANN implementations are currently hampered by the lack of efficient hardware synapses; a key component that every ANN requires in large numbers.
In the study, published in Nature Communications, the Southampton research team experimentally demonstrated an ANN that used memristor synapses supporting sophisticated learning rules in order to carry out reversible learning of noisy input data.
Memristors are electrical components that limit or regulate the flow of electrical current in a circuit and can remember the amount of charge that was flowing through it and retain the data, even when the power is turned off.
Lead author Dr Alex Serb, from Electronics and Computer Science at the University of Southampton, said: “If we want to build artificial systems that can mimic the brain in function and power we need to use hundreds of billions, perhaps even trillions of artificial synapses, many of which must be able to implement learning rules of varying degrees of complexity. Whilst currently available electronic components can certainly be pieced together to create such synapses, the required power and area efficiency benchmarks will be extremely difficult to meet -if even possible at all- without designing new and bespoke 'synapse components'.
“Memristors offer a possible route towards that end by supporting many fundamental features of learning synapses (memory storage, on-line learning, computationally powerful learning rule implementation, two-terminal structure) in extremely compact volumes and at exceptionally low energy costs. If artificial brains are ever going to become reality, therefore, memristive synapses have to succeed.”
Acting like synapses in the brain, the metal-oxide memristor array was capable of learning and re-learning input patterns in an unsupervised manner within a probabilistic winner-take-all (WTA) network. This is extremely useful for enabling low-power embedded processors (needed for the Internet of Things) that can process in real-time big data without any prior knowledge of the data.
Brain-inspired device to power artificial systems
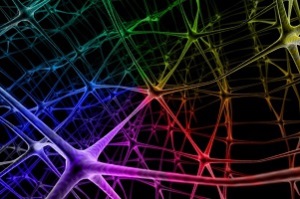
Artificial neural networks (ANNs) exhibit learning abilities and can perform tasks which are difficult for conventional computing systems, such as pattern recognition, on-line learning and classification. Practical ANN implementations are currently hampered by the lack of efficient hardware synapses; a key component that every ANN requires in large numbers.
In the study, published in Nature Communications, the Southampton research team experimentally demonstrated an ANN that used memristor synapses supporting sophisticated learning rules in order to carry out reversible learning of noisy input data.
Memristors are electrical components that limit or regulate the flow of electrical current in a circuit and can remember the amount of charge that was flowing through it and retain the data, even when the power is turned off.
Lead author Dr Alex Serb, from Electronics and Computer Science at the University of Southampton, said: “If we want to build artificial systems that can mimic the brain in function and power we need to use hundreds of billions, perhaps even trillions of artificial synapses, many of which must be able to implement learning rules of varying degrees of complexity. Whilst currently available electronic components can certainly be pieced together to create such synapses, the required power and area efficiency benchmarks will be extremely difficult to meet -if even possible at all- without designing new and bespoke 'synapse components'.
“Memristors offer a possible route towards that end by supporting many fundamental features of learning synapses (memory storage, on-line learning, computationally powerful learning rule implementation, two-terminal structure) in extremely compact volumes and at exceptionally low energy costs. If artificial brains are ever going to become reality, therefore, memristive synapses have to succeed.”
Acting like synapses in the brain, the metal-oxide memristor array was capable of learning and re-learning input patterns in an unsupervised manner within a probabilistic winner-take-all (WTA) network. This is extremely useful for enabling low-power embedded processors (needed for the Internet of Things) that can process in real-time big data without any prior knowledge of the data.
Views: 535
©ictnews.az. All rights reserved.Similar news
- The mobile sector continues its lead
- Facebook counted 600 million active users
- Cell phone testing laboratory is planned to be built in Azerbaijan
- Tablets and riders outfitted quickly with 3G/4G modems
- The number of digital TV channels will double to 24 units
- Tax proposal in China gets massive online feedback
- Malaysia to implement biometric system at all entry points
- Korea to build Green Technology Centre
- Cisco Poised to Help China Keep an Eye on Its Citizens
- 3G speed in Azerbaijan is higher than in UK
- Government of Canada Announces Investment in Green Innovation for Canada
- Electric cars in Azerbaijan
- Dominican Republic Govt Issues Cashless Benefits
- Spain raises €1.65bn from spectrum auction
- Camden Council boosts mobile security