



Date:08/11/18
A team of US researchers has found that artificial intelligence (AI) can improve the ability of brain imaging to detect Alzheimer’s earlier.
The deep learning algorithm created by the researchers achieved 100% sensitivity at detecting the disease an average of more than six years prior to the formal diagnosis.
A study describing the AI algorithm and its results has been published in Radiology.
Early diagnosis can be crucial for Alzheimer’s patients as treatments are more effective in the early stages of the disease. However, early diagnosis is challenging and some of the known signs of the disease, such as changes in metabolism affecting glucose uptake in the brain, can be difficult to recognise.
Dr Jae Ho Sohn from the Radiology & Biomedical Imaging Department at the University of California in San Francisco (UCSF) was the study’s co-author. He said: “Differences in the pattern of glucose uptake in the brain are very subtle and diffuse. People are good at finding specific biomarkers of disease, but metabolic changes represent a more global and subtle process.”
The study’s senior author is Dr Benjamin Franc from UCSF. He collaborated with Sohn and University of California, Berkeley undergraduate student Yiming Ding through the Big Data in Radiology (BDRAD) research group, a multidisciplinary team of physicians and engineers focusing on radiological data science.
Franc was interested in using deep learning to pinpoint the changes in brain metabolism that are predictive of Alzheimer’s disease.
The research team trained a deep learning algorithm on an imaging technology known as 18-F-fluorodeoxyglucose positron emission tomography (FDG-PET). FDG is a radioactive glucose compound which is injected into a patient’s blood if they undergo a FDG-PET scan. The PET scan can then measure FDG uptake in brain cells, which will indicate metabolic activity.
The researchers used data from the Alzheimer’s Disease Neuroimaging Initiative (ADNI), a multi-site study focused on clinical trials to improve the prevention and treatment of Alzheimer’s. The deep learning algorithm was trained on 90% of the dataset and then tested on the remaining 10%. The algorithm was able to teach itself metabolic patterns that correspond to Alzheimer’s disease.
Finally, the algorithm was used on an independent set of 40 imaging exams from 40 patients that it had never studied and achieved 100% sensitivity at detecting the disease an average of more than six years before a diagnosis.
Sohn said: “We were very pleased with the algorithm’s performance. It was able to predict every single case that advanced to Alzheimer’s disease.
“If we diagnose Alzheimer’s disease when all the symptoms have manifested, the brain volume loss is so significant that it’s too late to intervene. If we can detect it earlier, that’s an opportunity for investigators to potentially find better ways to slow down or even halt the disease process.”
The researchers now have plans to train the deep learning algorithm to look for patterns associated with the accumulation of beta-amyloid and tau proteins.
Artificial intelligence can predict Alzheimer’s years before diagnosis
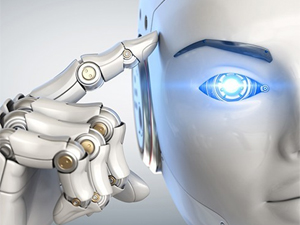
The deep learning algorithm created by the researchers achieved 100% sensitivity at detecting the disease an average of more than six years prior to the formal diagnosis.
A study describing the AI algorithm and its results has been published in Radiology.
Early diagnosis can be crucial for Alzheimer’s patients as treatments are more effective in the early stages of the disease. However, early diagnosis is challenging and some of the known signs of the disease, such as changes in metabolism affecting glucose uptake in the brain, can be difficult to recognise.
Dr Jae Ho Sohn from the Radiology & Biomedical Imaging Department at the University of California in San Francisco (UCSF) was the study’s co-author. He said: “Differences in the pattern of glucose uptake in the brain are very subtle and diffuse. People are good at finding specific biomarkers of disease, but metabolic changes represent a more global and subtle process.”
The study’s senior author is Dr Benjamin Franc from UCSF. He collaborated with Sohn and University of California, Berkeley undergraduate student Yiming Ding through the Big Data in Radiology (BDRAD) research group, a multidisciplinary team of physicians and engineers focusing on radiological data science.
Franc was interested in using deep learning to pinpoint the changes in brain metabolism that are predictive of Alzheimer’s disease.
The research team trained a deep learning algorithm on an imaging technology known as 18-F-fluorodeoxyglucose positron emission tomography (FDG-PET). FDG is a radioactive glucose compound which is injected into a patient’s blood if they undergo a FDG-PET scan. The PET scan can then measure FDG uptake in brain cells, which will indicate metabolic activity.
The researchers used data from the Alzheimer’s Disease Neuroimaging Initiative (ADNI), a multi-site study focused on clinical trials to improve the prevention and treatment of Alzheimer’s. The deep learning algorithm was trained on 90% of the dataset and then tested on the remaining 10%. The algorithm was able to teach itself metabolic patterns that correspond to Alzheimer’s disease.
Finally, the algorithm was used on an independent set of 40 imaging exams from 40 patients that it had never studied and achieved 100% sensitivity at detecting the disease an average of more than six years before a diagnosis.
Sohn said: “We were very pleased with the algorithm’s performance. It was able to predict every single case that advanced to Alzheimer’s disease.
“If we diagnose Alzheimer’s disease when all the symptoms have manifested, the brain volume loss is so significant that it’s too late to intervene. If we can detect it earlier, that’s an opportunity for investigators to potentially find better ways to slow down or even halt the disease process.”
The researchers now have plans to train the deep learning algorithm to look for patterns associated with the accumulation of beta-amyloid and tau proteins.
Views: 284
©ictnews.az. All rights reserved.Similar news
- Justin Timberlake takes stake in Facebook rival MySpace
- Wills and Kate to promote UK tech sector at Hollywood debate
- 35% of American Adults Own a Smartphone
- How does Azerbaijan use plastic cards?
- Imperial College London given £5.9m grant to research smart cities
- Search and Email Still the Most Popular Online Activities
- Nokia to ship Windows Phone in time for holiday sales
- Internet 'may be changing brains'
- Would-be iPhone buyers still face weeks-long waits
- Under pressure, China company scraps Steve Jobs doll
- Jobs was told anti-poaching idea "likely illegal"
- Angelic "Steve Jobs" loves Android in Taiwan TV ad
- Kinect for Windows gesture sensor launched by Microsoft
- Kindle-wielding Amazon dips toes into physical world
- Video game sales fall ahead of PlayStation Vita launch