



Date:17/07/18
As far as the Department of Defense is concerned, artificial intelligence is a team game, particularly where robots are concerned.
Researchers at the Army Research Laboratory (ARL), working with a team from Carnegie Mellon University, have taken another step toward the team concept, developing a new technique that enables robots to work on their own with only nominal human oversight.
In this case, a robotic mobile platform was able to autonomously navigate a battlefield-like environment using visual perception and a fairly small amount of data, performing the way its human counterparts expected it to operate without having to be guided along the way. The robot stayed along the side of a road and managed to move covertly, using buildings as cover, and got to where it was supposed to be, according to an ARL release.
The study furthers a primary goal in AI research–finding ways for machines to learn more quickly by watching examples and receiving feedback, rather than first being programmed or trained on every possible scenario. A robot performing reliably on its own doesn’t require constant input from a human controller or line-of-sight control. Instead, it creates a sense of trust between humans and machines that is seen as essential to working as a team.
"If a robot acts as a teammate, tasks can be accomplished faster and more situational awareness can be obtained," said ARL researcher Maggie Wigness, one of the co-authors of a paper on the research presented at the Institute of Electrical and Electronics Engineers’ International Conference on Robotics and Automation held in May in Brisbane, Australia. "Further, robot teammates can be used as an initial investigator for potentially dangerous scenarios, thereby keeping soldiers further from harm."
ARL, working with the University of Texas, Austin, is taking a similar approach with its Deep TAMER algorithm, with which they trained a machine by offering relatively few examples and then provided a steady stream of positive or negative feedback when the machine tried the task on its own. In one case, a machine was able to beat human experts at the old video game Atari Bowling after only 15 minutes of training.
With its ground robot, ARL’s team used a reinforcement technique known as inverse optimal control, or inverse reinforcement learning, which essentially uses a reward function to encourage the most appropriate learned behavior, ARL said. A human demonstrated the optimal way to drive through a setting, thus demonstrating the behavior to be learned, with relation to features such as grass, roads, and buildings. Eventually, the robot learns to activate the most appropriate traversal behavior depending on an individual setting.
In addition to improving the performance of ground robotic systems in the military, ARL’s unique parameters–a contested, noisy, unstructured environment–could also benefit robotics research overall. ARL’s research is funded by the Army’s Robotics Collaborative Technology Alliance, or RCTA, which supports combined efforts of government, industry, and academia with regard to ground robotics.
Whether on the ground, at sea, or in the air, AI-powered robotics is a key element of DoD’s Third Offset Strategy for military improvements in the coming years. Other projects the services are working on include robotic combat vehicles, munitions recovery, and soldier training, among many others.
Army Is Teaching AI Robots the Team Concept
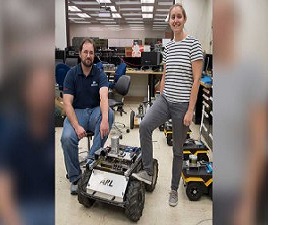
Researchers at the Army Research Laboratory (ARL), working with a team from Carnegie Mellon University, have taken another step toward the team concept, developing a new technique that enables robots to work on their own with only nominal human oversight.
In this case, a robotic mobile platform was able to autonomously navigate a battlefield-like environment using visual perception and a fairly small amount of data, performing the way its human counterparts expected it to operate without having to be guided along the way. The robot stayed along the side of a road and managed to move covertly, using buildings as cover, and got to where it was supposed to be, according to an ARL release.
The study furthers a primary goal in AI research–finding ways for machines to learn more quickly by watching examples and receiving feedback, rather than first being programmed or trained on every possible scenario. A robot performing reliably on its own doesn’t require constant input from a human controller or line-of-sight control. Instead, it creates a sense of trust between humans and machines that is seen as essential to working as a team.
"If a robot acts as a teammate, tasks can be accomplished faster and more situational awareness can be obtained," said ARL researcher Maggie Wigness, one of the co-authors of a paper on the research presented at the Institute of Electrical and Electronics Engineers’ International Conference on Robotics and Automation held in May in Brisbane, Australia. "Further, robot teammates can be used as an initial investigator for potentially dangerous scenarios, thereby keeping soldiers further from harm."
ARL, working with the University of Texas, Austin, is taking a similar approach with its Deep TAMER algorithm, with which they trained a machine by offering relatively few examples and then provided a steady stream of positive or negative feedback when the machine tried the task on its own. In one case, a machine was able to beat human experts at the old video game Atari Bowling after only 15 minutes of training.
With its ground robot, ARL’s team used a reinforcement technique known as inverse optimal control, or inverse reinforcement learning, which essentially uses a reward function to encourage the most appropriate learned behavior, ARL said. A human demonstrated the optimal way to drive through a setting, thus demonstrating the behavior to be learned, with relation to features such as grass, roads, and buildings. Eventually, the robot learns to activate the most appropriate traversal behavior depending on an individual setting.
In addition to improving the performance of ground robotic systems in the military, ARL’s unique parameters–a contested, noisy, unstructured environment–could also benefit robotics research overall. ARL’s research is funded by the Army’s Robotics Collaborative Technology Alliance, or RCTA, which supports combined efforts of government, industry, and academia with regard to ground robotics.
Whether on the ground, at sea, or in the air, AI-powered robotics is a key element of DoD’s Third Offset Strategy for military improvements in the coming years. Other projects the services are working on include robotic combat vehicles, munitions recovery, and soldier training, among many others.
Views: 671
©ictnews.az. All rights reserved.Similar news
- The mobile sector continues its lead
- Facebook counted 600 million active users
- Cell phone testing laboratory is planned to be built in Azerbaijan
- Tablets and riders outfitted quickly with 3G/4G modems
- The number of digital TV channels will double to 24 units
- Tax proposal in China gets massive online feedback
- Malaysia to implement biometric system at all entry points
- Korea to build Green Technology Centre
- Cisco Poised to Help China Keep an Eye on Its Citizens
- 3G speed in Azerbaijan is higher than in UK
- Government of Canada Announces Investment in Green Innovation for Canada
- Electric cars in Azerbaijan
- Dominican Republic Govt Issues Cashless Benefits
- Spain raises €1.65bn from spectrum auction
- Camden Council boosts mobile security